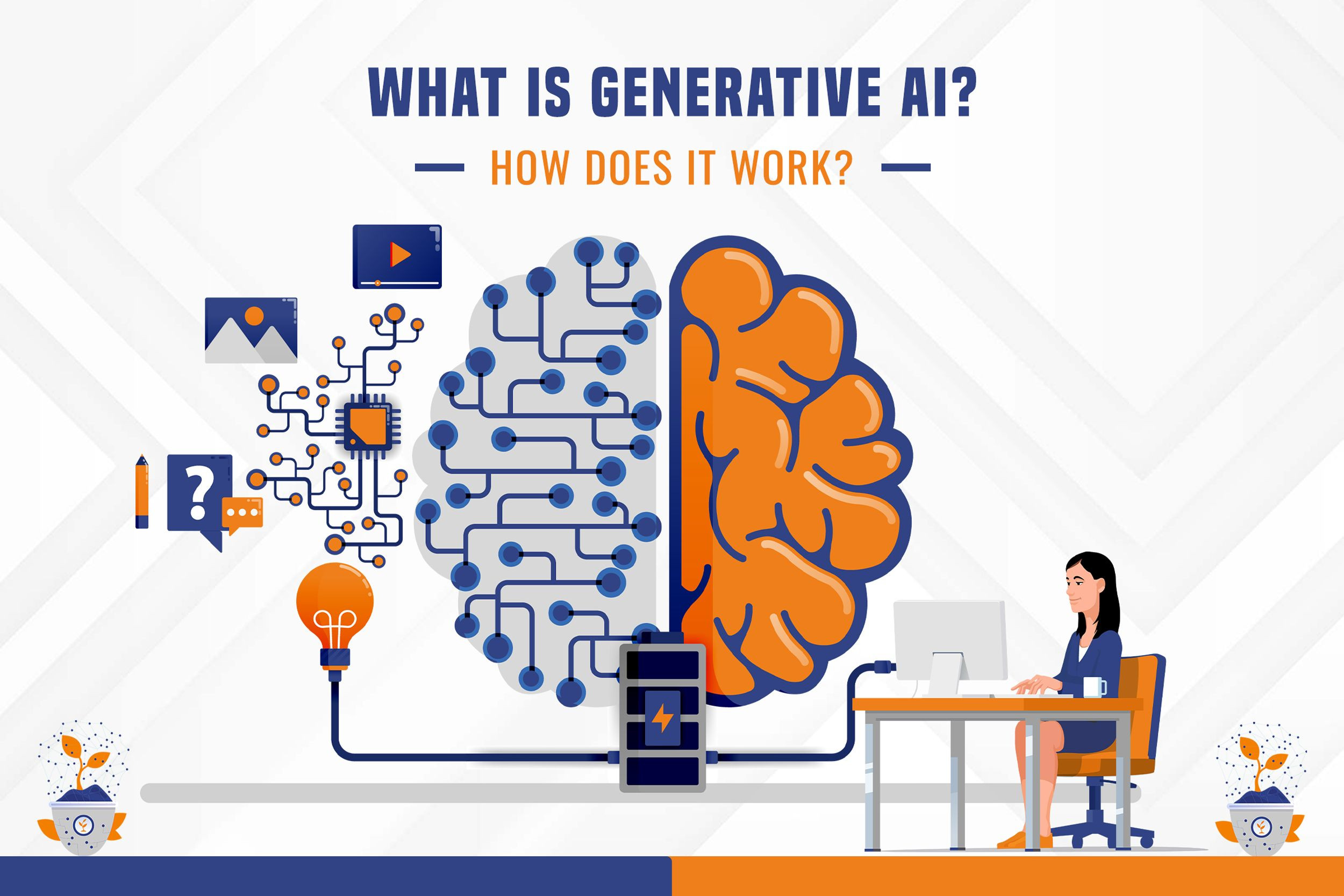
What is Generative AI? How does it Work?
In the realm of artificial intelligence, Generative AI stands as a captivating and transformative concept. It empowers machines to generate content, be it text, images, music, or even entire pieces of art, autonomously. This cutting-edge technology has opened up new horizons for creativity, innovation, and problem-solving. So let’s delve deep into the fascinating world of Generative AI, understanding what it is, how it works, and its various applications.
What is Generative AI? How Does Generative AI Work?
Generative AI refers to a subset of artificial intelligence that focuses on creating or generating content that is typically associated with human creativity and ingenuity. Unlike conventional AI models that are designed for specific tasks, such as image recognition or natural language processing, Generative AI systems have the unique ability to generate novel content autonomously.
The core principle behind Generative AI is to enable machines to Technical Learning and understand patterns and structures in data so they can produce new data that fits within those patterns. This is achieved through the use of neural networks, a technology inspired by the structure and function of the human brain.
Generative AI systems are primarily powered by deep learning algorithms, particularly Generative Adversarial Networks (GANs) and Recurrent Neural Networks (RNNs). Let's delve into how these technologies work:
Generative Adversarial Networks (GANs):
GANs consist of two neural networks, the generator, and the discriminator, that work in opposition. Here's how they function:
Generator:
The generator network takes random noise as input and generates data (e.g., images, text) that mimics the patterns and structures present in the training data. Initially, the generated content is random and unrecognizable.
Discriminator:
The discriminator network's role is to distinguish between real data from the training set and the synthetic data produced by the generator. It learns to identify subtle differences and inconsistencies.
The training process involves a constant interplay between these two networks:
- The generator tries to improve its output to fool the discriminator by generating content that looks increasingly realistic.
- The discriminator becomes better at distinguishing between real and fake data.
- This adversarial process continues until the generated content is nearly indistinguishable from real data.
- GANs have been used to create remarkably convincing deepfake videos, and realistic images, and even generate text that closely resembles human writing.
Recurrent Neural Networks (RNNs):
RNNs are another key technology in Generative AI, particularly when it comes to generating sequences of data, such as text and music. Unlike traditional neural networks, RNNs have feedback loops that allow them to maintain information about previous inputs, making them suitable for tasks involving sequential data.
Here's how RNNs work:
Input:
RNNs take a sequence of input data, such as a sentence of words or a musical melody, one element at a time.
Hidden State:
The network maintains a hidden state that stores information about the previous inputs it has processed.
Output:
At each time step, the RNN generates an output based on the current input and the hidden state. This output can be a prediction, the next element in a sequence, or any other desired output.
Feedback Loop:
The hidden state is updated at each time step based on the current input and the previous hidden state. This allows RNNs to capture dependencies and patterns in sequential data.
RNNs have been employed in natural language generation, chatbots, and even composing music. They excel at tasks where the order and context of data are crucial in a business undergoing Technical Transformation.
Applications of Generative AI
Generative AI has a wide range of applications across various domains, revolutionizing how we create, innovate, and solve problems. Here are some notable applications:
Content Generation:
Generative AI can create textual content, images, videos, and music. It's used in content creation for marketing, entertainment, and design industries. For instance, chatbots can generate human-like responses, and AI algorithms can create artwork and music compositions.
Deepfake Technology:
GANs have raised concerns due to their ability to create convincing deepfake videos, which can be used for malicious purposes. However, they also have legitimate applications in video editing, special effects, and virtual reality.
Text Generation:
Natural language generation models, like OpenAI's GPT series, can generate coherent and contextually relevant text. They are used in chatbots, content creation, and even assisting writers in generating text for Technical Learning.
Drug Discovery:
Generative AI is making strides in drug discovery by predicting molecular structures and generating new drug candidates. This accelerates the drug development process and has implications for healthcare and pharmaceuticals.
Art and Creativity:
Generative AI is used in the creation of digital art, where it can produce novel and unique pieces based on artistic styles and influences. This intersects with the world of generative art.
Language Translation:
Generative models are used for language translation, enabling more accurate and context-aware translations. This has broad applications in international communication and business.
Autonomous Vehicles:
Generative AI helps self-driving cars navigate complex environments by generating real-time predictions and decisions based on sensor data.
Finance:
In finance, Generative AI is used for forecasting stock prices, generating financial reports, and detecting fraudulent transactions.
Challenges and Ethical Considerations
While Generative AI has immense potential, it also presents several challenges and ethical considerations:
Bias and Fairness:
Generative models can inherit biases present in their training data, leading to unfair or discriminatory outputs. Addressing bias in AI systems is crucial to ensure fairness and equity.
Misuse:
Massive Technical Transformation has negative effects too. The ability to create highly convincing deepfakes and fake content raises concerns about misinformation and malicious use. Regulation and safeguards are essential.
Data Privacy:
Generative AI systems can be used to generate realistic personal information, raising concerns about data privacy and identity theft.
Ethical Dilemmas:
As AI generates content that is increasingly indistinguishable from human-created content, it raises ethical questions about authorship, authenticity, and creative ownership.
Overreliance on AI:
There's a risk of overreliance on AI-generated content, potentially diminishing human creativity and innovation.
Final Words
Generative AI represents a remarkable frontier in artificial intelligence, empowering machines to exhibit creative and autonomous behavior. With technologies like GANs and RNNs, it can produce text, images, music, and more that are virtually indistinguishable from human creations. While Generative AI has made significant strides, it also poses ethical challenges, and careful consideration is needed to ensure responsible and equitable use. As this field continues to evolve, it holds the promise of transforming industries, pushing the boundaries of creativity, and redefining what is possible in the world of AI.