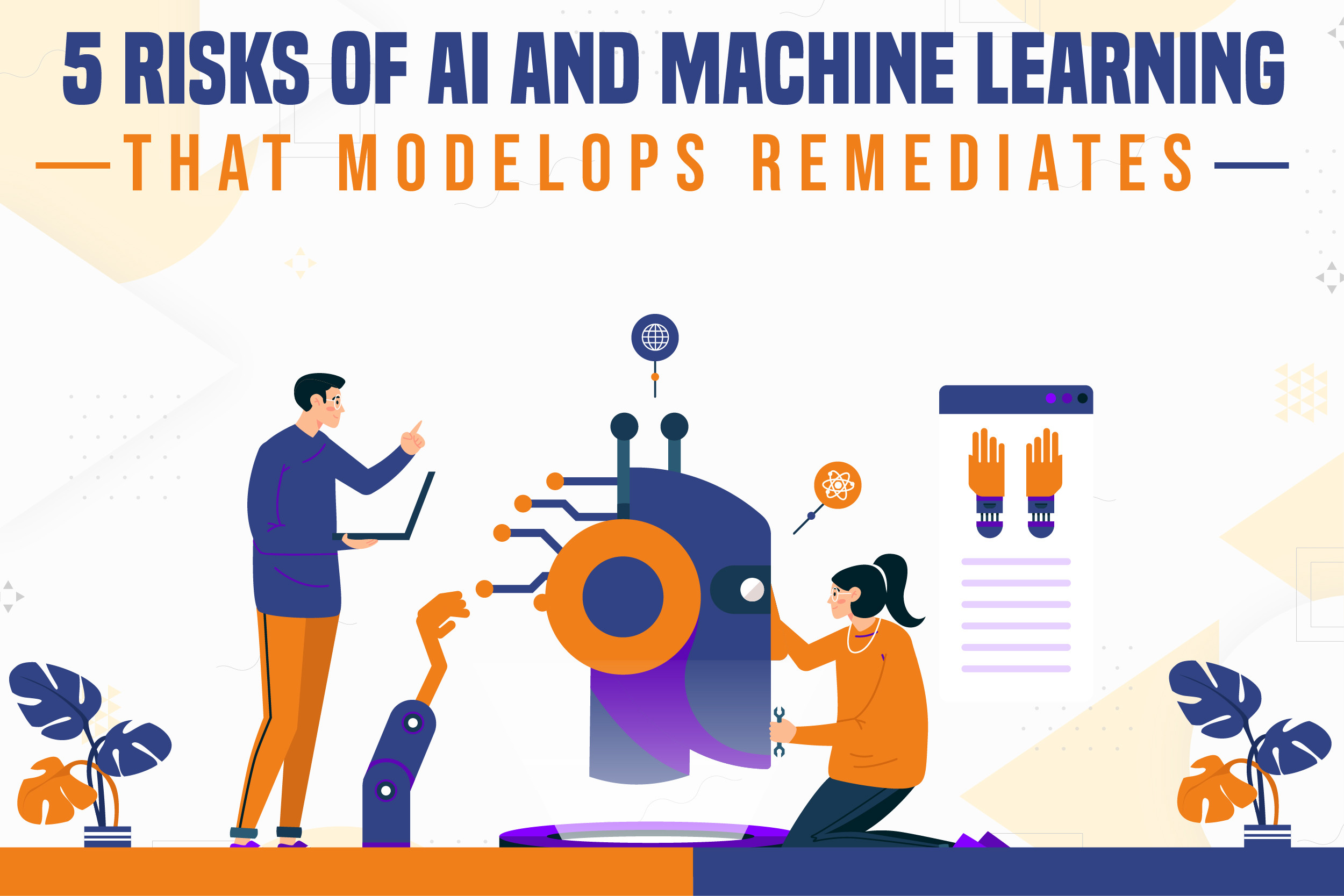
5 Risks Of AI And Machine Learning That Modelops Remediates
Artificial Intelligence (AI) and Machine Learning (ML) are rapidly changing the landscape of various industries, bringing a lot of benefits, but also introducing new risks and challenges. ModelOps is a set of best practices and tools for managing the end-to-end lifecycle of AI models, from development to deployment and beyond. It helps to mitigate the risks of AI and ML by improving the reliability and accuracy of models. In this article, we will explore the five main risks of AI and ML and how ModelOps can help to remediate them. But first, let’s have a brief idea of AI and ML.
AI And ML; A Brief Concept
Artificial Intelligence (AI) and Machine Learning (ML) are fields of computer science that focus on the development of intelligent systems that can perform tasks that typically require human intelligence, such as understanding natural language, recognizing images, and making predictions. AI and ML are transforming the way we live and work, enabling computers to perform tasks that were previously only possible for humans to do.
ML is a subset of AI that focuses on the development of algorithms that can learn from data and make predictions or decisions without being explicitly programmed to do so.
AI and ML are being applied to a wide range of industries, including healthcare, finance, and transportation, to improve efficiency, accuracy, and customer experience. AI and ML are also playing a critical role in solving some of the world's most pressing challenges, such as reducing energy consumption, improving medical diagnoses, and fighting climate change. Receiving Technical Training in these fields has become crucial more than ever thus.
Risks And Solutions Of AI And ML By Modelops
Risks of AI and ML can range from data bias, model performance degradation, lack of interpretability, security threats, and ethical issues. AI models are only as good as the data they are trained on, and if the data is biased, the model will also be biased. This can lead to unintended consequences and discriminatory outcomes.
ModelOps can help mitigate these risks by ensuring that AI models are developed, deployed, and monitored responsibly and sustainably. Let’s delve into these risks and their management in more detail:
1. Building Models With An Absence Of Risk Management Strategy
One of the biggest risks in AI and ML is the lack of a risk management strategy when developing models. This can result in models that are unreliable, inaccurate, and potentially harmful. Without a risk management strategy, organizations may overlook important considerations such as data bias, performance degradation, interpretability, security, and ethical issues. This can lead to unintended consequences and hurt the credibility of AI and ML.
To mitigate this risk, it is important to have a structured approach to developing AI and ML models that includes a risk management strategy. This strategy should take into account the potential risks associated with each step of the model development process, from data collection and preparation to deployment and monitoring. It should also outline the steps that need to be taken to address those risks, such as verifying the quality of the data, conducting thorough performance evaluations, and incorporating interpretability and fairness considerations.
Having a robust risk management strategy in place can help organizations develop AI and ML models that are reliable, accurate, and safe to use. It can also help to build trust in AI and ML, and enable organizations to take full advantage of the benefits that these technologies can bring.
2. Model Performance Degradation Over Time
This occurs when the performance of a model declines as a result of changes in the data distribution or the environment in which it is deployed. For example, a model that was trained on data collected in one geographic region may not perform as well when deployed in a different region with different data patterns.
To mitigate this risk, it is important to have a continuous monitoring and evaluation process in place. This process should regularly assess the performance of the model and detect any degradation in real time. If degradation is detected, the model should be updated or retrained on more recent data to improve its accuracy.
Additionally, organizations should consider using ModelOps to manage the end-to-end lifecycle of their AI and ML models. ModelOps can help to monitor the performance of models over time and detect any degradation, allowing organizations to take proactive steps to address it.
3. Enhancing Maintenance With Domain-specific And Duplicate Models
The development of AI and ML models is about the challenge of increasing maintenance costs due to the proliferation of duplicate and domain-specific models. As organizations adopt AI and ML technologies, they may create multiple models to solve similar problems, leading to a proliferation of models that are difficult to manage and maintain. Additionally, domain-specific models may be created for each use case, adding to the maintenance burden.
To mitigate this risk, organizations should adopt a centralized model management strategy that includes guidelines for creating and maintaining models. This should include a review process to ensure that new models are aligned with existing models and do not duplicate existing capabilities. In addition, organizations should consider using a platform for model management that provides a centralized repository for all models, allowing for easy discovery, reuse, and collaboration.
To keep abreast of the Technical Transformation it's crucial to upskill your workforce. Get in touch with us for an immersive and result-driven training experience.
4. Bureaucratic Review Boards
In many organizations, the deployment of AI and ML models is subject to review by multiple stakeholders, including legal, privacy, and security teams, who may have different priorities and objectives. This can result in a lengthy and complex review process that slows down the deployment of models and creates bottlenecks.
To mitigate this risk, organizations should establish a clear and streamlined review process for AI and ML models that are aligned with business objectives and enables rapid deployment. This should involve close collaboration between all stakeholders, including legal, privacy, and security teams, to ensure that models are reviewed and approved promptly. Additionally, organizations should consider using automated tooling to manage the review process and reduce the administrative burden associated with manual reviews.
By streamlining the review process and reducing bureaucracy, organizations can ensure that AI and ML models are deployed quickly and efficiently, improving the overall speed and effectiveness of their AI and ML initiatives Technical Training Programs. nowadays are structured and devised around these topics to provide a better grip on the technologies to the employees.
5. Unable To track Models For Operational Issues And Data Drift
As models are deployed in production, they may encounter changes in the data distribution and operational environment that can impact their performance. For example, a model that was trained on a specific data set may no longer perform well as the data distribution changes over time.
To mitigate this risk, it is important to have a continuous monitoring and evaluation process in place that identifies any data drift or operational issues and takes proactive steps to address them. This is why providing Technical Training to the team is important to expertly handle those issues. This process should involve regularly monitoring the performance of models and comparing it to expected outcomes, as well as detecting and addressing any issues in real-time.
Moreover, organizations should consider using ModelOps to manage the end-to-end lifecycle of their AI and ML models. ModelOps can help to monitor the performance of models over time and detect any data drift or operational issues, allowing organizations to take proactive steps to address them.
Final Words
Thus, the development of AI and ML models comes with several risks that can impact the accuracy, reliability, and performance of models. To mitigate these risks, organizations should adopt best practices for ModelOps, including a centralized model management strategy, a streamlined review process, and continuous monitoring and evaluation. By doing so, organizations can ensure that their AI and ML models perform well and deliver accurate results, enabling them to achieve their desired business outcomes.