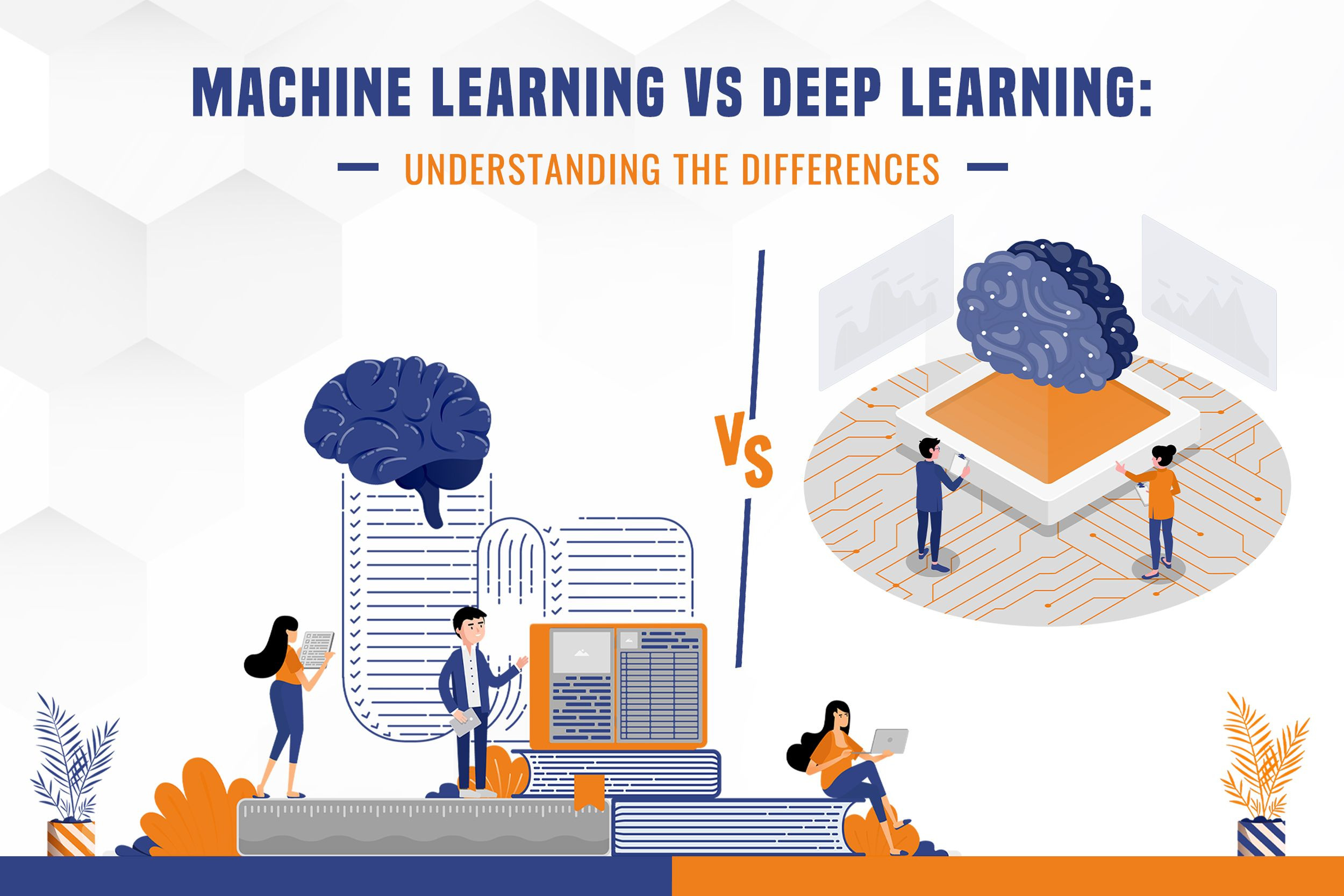
Machine Learning vs Deep Learning: Understanding the Differences
In the world of artificial intelligence and data science, terms like Machine Learning and Deep Learning often surface. While they are closely related, they possess distinct characteristics that set them apart. In this article, we'll delve into the intricacies of Machine Learning vs. Deep Learning and how they differ from each other, shedding light on their definitions, applications, advantages, and limitations.
Machine Learning vs Deep Learning: An In-Depth Comparison
Machine Learning Defined
Machine Learning utilizes algorithms to empower computers to learn from data, enabling them to make predictions or decisions. It's a subset of artificial intelligence that focuses on creating systems that can adapt and improve over time without being explicitly programmed.
Deep Learning Defined
Deep Learning, as a subset of Machine Learning, emulates the intricate neural networks of the human brain. It employs artificial neural networks with multiple layers to process data and extract intricate patterns. Deep Learning models have shown remarkable performance in tasks such as image recognition, natural language processing, and more, which is why Technical Training Courses on these skills are extremely rewarding for one’s career.
Key Differences
Aspect |
Machine Learning |
Deep Learning |
Complexity |
Less complex |
Highly complex |
Feature Extraction |
Manual feature extraction required |
Automatic feature extraction
|
Data Dependencies |
Moderate data dependencies |
High data dependencies |
Performance |
Good for small datasets |
Excellent performance on large datasets |
Training Time |
Faster training time |
Longer training time |
Interpretability |
More interpretable |
Less interpretable |
Applications of Machine Learning and Deep Learning
Machine Learning Applications
Machine Learning finds applications in various domains:
- Predictive Analytics: ML models analyze historical data to make predictions about future outcomes.
- Recommendation Systems: E-commerce platforms use ML to suggest products based on user preferences.
- Fraud Detection: ML algorithms identify fraudulent activities by spotting unusual patterns.
- Medical Diagnostics: ML aids doctors in diagnosing diseases by analyzing patient data.
Deep Learning Applications
Deep Learning excels in tasks demanding intricate pattern recognition:
- Image and Speech Recognition: Deep Learning powers facial recognition and voice assistants.
- Natural Language Processing: It enables sentiment analysis, language translation, and chatbots.
- Autonomous Vehicles: Deep Learning contributes to self-driving car technology.
- Drug Discovery: It accelerates drug discovery by predicting molecular interactions.
Advantages and Limitations
Advantages of Machine Learning
- Interpretability: ML models are often more understandable, allowing humans to grasp decision-making processes. It is being taught in many Technical Training Programs.
- Resource Efficiency: They require fewer computational resources compared to deep neural networks.
- Quick Implementation: Machine Learning models can be implemented faster due to their simplicity.
Limitations of Machine Learning
- Feature Engineering: Manual feature engineering can be time-consuming and may not capture complex patterns.
- Scalability: Performance may decrease as datasets grow larger and more complex.
- Limited Complexity: ML models may struggle with tasks requiring high levels of complexity.
Advantages of Deep Learning
- Complex Pattern Recognition: Deep Learning excels in tasks where subtle patterns need to be detected.
- Automated Feature Learning: Deep Learning models automatically learn relevant features from raw data.
- State-of-the-Art Performance: It achieves state-of-the-art performance in various fields.
Limitations of Deep Learning
- Data Dependency: Deep Learning models require substantial amounts of data for effective training and Technical Learning.
- Computationally Intensive: Training deep neural networks demands significant computational resources.
- Interpretability Challenge: Understanding decisions made by deep models can be challenging.
Machine Learning vs Deep Learning in Real-world Scenarios
Financial Fraud Detection
Machine Learning Approach
The Machine Learning approach is adept at identifying financial fraud by meticulously analyzing transaction data for irregular patterns. For instance, it can promptly recognize transactions that veer from an individual's established spending habits, enabling it to effectively flag potential instances of fraud. This technique empowers financial institutions to swiftly detect and mitigate fraudulent activities, safeguarding both users and their financial assets. As a result, intensive Technical Learning is no longer an option but has grown to be a necessity these days.
Deep Learning Approach
The Deep Learning approach leverages its remarkable capability to discern intricate patterns, enabling it to uncover subtle indicators of fraud that conventional methods might overlook. This advanced technique excels at detecting anomalies and irregularities within financial data, even those that are deeply embedded or less apparent. By harnessing the power of deep neural networks, this approach offers a heightened level of accuracy in identifying fraudulent activities, thereby enhancing the overall security measures implemented by financial institutions.
Final Words
In the dynamic landscape of artificial intelligence, understanding the differences between Machine Learning and Deep Learning is crucial. Both approaches have their unique advantages and limitations, making them suitable for distinct applications. Machine Learning's interpretability and resource efficiency make it ideal for various scenarios, while Deep Learning's complex pattern recognition is invaluable for tasks requiring intricate analysis. As technology advances, harnessing the power of both paradigms will lead to innovative breakthroughs across industries.